AMD Is Losing Ground to NVIDIA in the AI Chip War
The race for dominance in the AI hardware market is heating up, and NVIDIA is pulling ahead by a wide margin. Once considered a neck-and-neck competitor in the GPU space, AMD now finds itself struggling to keep pace—particularly when it comes to AI and data center solutions. Recent revenue results, software adoption rates, and hardware performance benchmarks all point to one hard truth: AMD is falling behind in a space NVIDIA has aggressively secured.
NVIDIA’s AI Empire: Market Share and Strategic Vision
NVIDIA currently commands over 80% of the AI GPU market, a staggering lead driven not only by powerful hardware like the H100 Tensor Core GPUs but also by its unmatched software ecosystem. The CUDA platform has become the de facto standard for AI developers, researchers, and enterprises. From academia to billion-dollar tech firms, everyone building AI models at scale is likely doing so on CUDA-compatible hardware.
This software moat is critical. CUDA has had a decade-plus head start, and it enables deep integration with popular AI frameworks like PyTorch and TensorFlow—something AMD has yet to fully replicate.
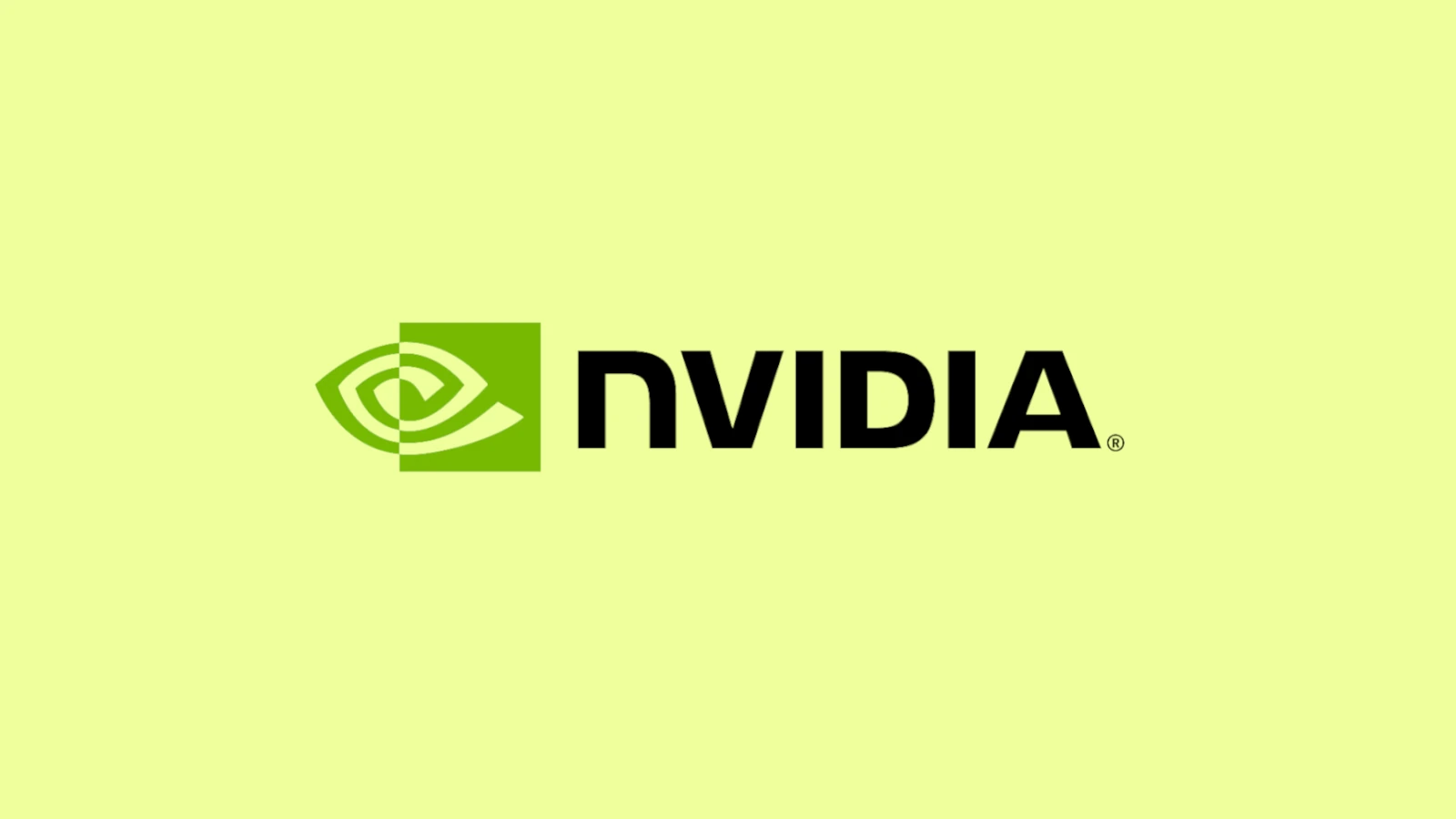
AMD’s Struggles: Late To the AI Software Game
While AMD’s Instinct MI300X accelerators have shown promising specs on paper, they’ve underwhelmed in early real-world adoption and performance benchmarks. Analysts and insiders note that AMD’s lack of a mature AI software platform has been one of its biggest weaknesses. The company has historically focused on raw hardware specs, but in AI, software tooling and ease of integration can matter just as much—if not more.
In Q1 2025, AMD’s AI revenue numbers fell short of Wall Street expectations, triggering a stock dip of over 10%. The contrast with NVIDIA couldn’t be starker: NVIDIA posted record-breaking earnings from AI data center sales and has orders booked out months in advance, even amid supply constraints.
Key Challenges AMD Faces in the AI Market
- Performance Gap: NVIDIA’s Hopper (H100) and upcoming Blackwell architecture are optimized for AI training and inference workloads, giving them a performance edge over AMD’s MI series.
- Ecosystem Disadvantage: NVIDIA’s CUDA dominance means AMD has to convince developers to switch or build from scratch using ROCm, its own platform, which still lacks ecosystem maturity.
- Customer Mindshare: Enterprise customers have grown accustomed to NVIDIA’s proven reliability, customer support, and software stack—all of which AMD must work hard to match.
What AMD Is Doing to Fight Back
To its credit, AMD is not sitting idle. The company is making a serious push into AI, and here are the strategic moves it’s made recently:
- Acquisitions for AI Expertise: AMD has acquired startups like Nod.ai and Silo AI to boost its in-house software development talent and expand its AI framework capabilities.
- New Hardware Rollouts: AMD is preparing to launch the MI325X and MI350 series GPUs, which are expected to offer better price-to-performance ratios and improved integration with industry-standard ML libraries.
- Data Center Push: The acquisition of ZT Systems, a key player in cloud and hyperscale server manufacturing, signals AMD’s intention to capture more of the data center market that NVIDIA currently dominates.
Financial Divergence
The financial gap between AMD and NVIDIA is also growing, especially in AI-related segments. While AMD reported $23.6 billion in total revenue in 2022, NVIDIA reported $26.9 billion in the same year—but a large and rapidly growing portion of NVIDIA’s income is now AI-focused. That disparity has widened significantly in 2024 and early 2025 as NVIDIA reaps the rewards of early bets on generative AI and LLMs (large language models).
The Bottom Line
AMD is in the fight, but it’s swinging uphill. The company still plays an important role as a competitive check on NVIDIA’s pricing power and offers compelling alternatives in other segments like consumer graphics and CPUs. But in AI hardware, it’s NVIDIA’s game to lose—for now.
Unless AMD’s software investments and next-gen hardware efforts pay off quickly, the gap in both market share and perception may continue to grow. For now, NVIDIA remains the undisputed leader in the AI arms race.
Key Takeaways
- NVIDIA leads the AI chip race through early investments in both hardware and software solutions.
- AMD’s failure to focus on software development has created a competitive disadvantage despite having capable hardware.
- Market share differences between the companies continue to grow, affecting AMD’s financial performance and stock value.
Market Dynamics and Competitive Landscape
The AI chip market has evolved into a battlefield where NVIDIA currently maintains a commanding lead over AMD. This dominance stems from NVIDIA’s early investments in AI-specific hardware and software ecosystems, while AMD struggles to catch up despite recent efforts to gain market share.
AI Chip Revenue and Market Share
NVIDIA controls approximately 80% of the AI chip market, with its data center revenue reaching record levels quarter after quarter. In contrast, AMD holds less than 10% market share despite being the second-largest player in the GPU space. This gap widened after the AI boom started in late 2022.
The financial numbers tell a clear story. NVIDIA’s data center revenue grew by over 400% year-over-year in recent quarters, while AMD’s AI-related revenue, though growing, remains a fraction of its competitor’s. Investors have noticed this disparity, with NVIDIA’s stock price increasing dramatically compared to AMD’s more modest gains.
Key factors behind this market share difference include:
- Software ecosystem advantage: NVIDIA’s CUDA platform has become the industry standard
- First-mover advantage: Years of AI investment before the current boom
- Production capacity: More chips available when demand surged
Strategic Partnerships and Industry Adoption
NVIDIA has secured partnerships with nearly all major AI players including OpenAI, Microsoft, and Meta. These relationships have created a powerful network effect. Most AI training workloads run on NVIDIA hardware by default.
AMD has made progress with some hyperscalers, particularly Microsoft, which uses AMD chips in some Azure AI offerings. However, developer preference remains strongly in NVIDIA’s favor. A recent survey showed that over 90% of AI developers prefer NVIDIA’s development environment over alternatives.
The adoption gap is especially visible in large language model training, where most major models like GPT-4 were trained primarily on NVIDIA hardware. This creates a feedback loop: more models optimized for NVIDIA means more developers choose NVIDIA, which leads to more optimization.
Recent Performance and Technological Advances
AMD’s latest MI300 accelerators show promising performance metrics and could narrow the gap with NVIDIA’s H100 chips. Early benchmarks suggest AMD might offer better performance-per-dollar in specific workloads.
However, NVIDIA continues advancing its technology with the upcoming Blackwell architecture promising significant performance gains. These chips are expected to be 30x faster than previous generations for certain AI tasks.
The software gap remains the biggest challenge for AMD. Despite creating ROCm as an alternative to CUDA, adoption has been slow. Many AI frameworks still run better on NVIDIA hardware due to years of optimization.
AMD is making strategic investments to catch up, including:
- Improving developer tools
- Enhancing AI-specific hardware features
- Building relationships with key AI research labs
But NVIDIA keeps moving the target with advances in technologies like DLSS and specialized AI inference solutions.
Technical Analysis and Industry Implications
The competition between AMD and NVIDIA in the AI chip market shows stark differences in technical capabilities and market positioning. These differences have significant implications for data centers, developers, and investors alike.
Impacts on Data Centers and Developers
NVIDIA’s dominance in AI chips has forced data centers to adapt their infrastructure to accommodate the company’s GPUs. This creates a technical lock-in effect that makes it difficult for competitors like AMD to gain traction. Data centers that have already invested heavily in NVIDIA’s architecture face high switching costs.
For developers, NVIDIA’s CUDA software ecosystem remains a major advantage. AMD’s ROCm platform, while improving, lacks the same level of support and optimization. This software gap matters as much as hardware performance when developers choose platforms.
GPU prices range from $20,000 to $40,000 per unit for data center use. While AMD offers better pricing, NVIDIA’s technical advantages and established developer ecosystem justify the premium for many customers.
Custom Chips and In-House Development
Large tech companies are increasingly developing their own custom AI chips to reduce dependence on NVIDIA. This trend could benefit AMD, which might be more willing to collaborate on custom designs.
ARM-based designs are gaining popularity for AI workloads. Companies like Amazon and Google have created custom chips based on ARM architecture to optimize for specific AI tasks.
AMD’s approach to custom chip development appears more flexible than NVIDIA’s. This could help AMD capture market share in specific segments where customization is valued over raw performance.
Custom Chip Development Approaches:
| Company | Approach | Key Advantage |
|---------|----------|---------------|
| NVIDIA | Highly integrated ecosystem | Performance optimized |
| AMD | More flexible partnerships | Customization options |
Investor Perspective and Future Outlook
AMD’s projected target for AI accelerators is $500 billion by 2028. Even capturing 10% would mean $75 billion in annual revenue, a massive growth from current figures.
Investors should note that AMD’s P/E multiple has declined substantially, potentially indicating better value. This contrasts with NVIDIA’s high valuation that assumes continued market dominance.
AMD’s $5.5 billion Instinct GPU forecast for 2024 remains small compared to NVIDIA’s quarterly AI chip revenue. However, AMD shares dropped 8% recently when AI chip revenue missed analyst expectations.
Forward-looking models like DeepSeek could increase demand for high-performance computing. Whether AMD can capture this demand depends on technical improvements and developer adoption of their platforms.
Frequently Asked Questions
The AI computing race between AMD and NVIDIA involves several technical, strategic, and market factors. NVIDIA currently holds a strong lead in AI hardware, but AMD continues to develop competitive offerings.
What are the key factors contributing to NVIDIA’s dominance over AMD in the AI sector?
NVIDIA gained its edge through early investment in CUDA, its proprietary parallel computing platform. This head start allowed NVIDIA to build a rich ecosystem of AI software tools and libraries.
The company also designed specialized hardware like Tensor Cores specifically for AI workloads. These cores speed up matrix calculations essential for machine learning.
NVIDIA’s partnerships with major cloud providers and research institutions further cemented its position. These relationships helped make NVIDIA GPUs the standard for AI development.
How do AMD’s GPUs compare to NVIDIA’s in terms of AI and machine learning performance?
AMD’s GPUs typically offer competitive raw computing power but lag in AI-specific optimizations. NVIDIA’s architecture is more efficient for the specific workloads used in deep learning.
Software support remains a key difference. NVIDIA’s CUDA platform has broader adoption than AMD’s ROCm, limiting AMD’s appeal to AI developers.
Recent benchmarks show AMD making progress, but NVIDIA still leads in most AI training and inference tasks by significant margins.
What strategic moves is AMD making to improve its position in the artificial intelligence market?
AMD is investing heavily in its ROCm software platform to improve compatibility with popular AI frameworks. This addresses a major barrier to adoption for AI developers.
The company has focused on creating more specialized AI hardware, including the MI300 accelerators. These chips aim to compete directly with NVIDIA’s data center offerings.
AMD is also leveraging its strong position in CPUs to create combined CPU-GPU solutions for AI workloads, offering potential cost and efficiency advantages.
How do investors view AMD’s potential to compete with NVIDIA in the AI field?
Investors remain cautious about AMD’s AI prospects despite enthusiasm for its overall business. This caution reflects NVIDIA’s entrenched position and technology advantages.
Some investment analysts see potential in AMD’s lower pricing strategy, which could help it gain market share. However, NVIDIA’s recent stock performance suggests the market still favors the leader.
The massive selloff in NVIDIA’s stock following news about competitors like DeepSeek AI shows that investors are watching for signs of change in the competitive landscape.
What are the specific technological advancements that give NVIDIA an edge in AI applications compared to AMD?
NVIDIA’s Tensor Cores provide specialized matrix multiplication capabilities critical for deep learning. These purpose-built components significantly outperform general-purpose computing for AI tasks.
The company’s interconnect technologies like NVLink allow for more efficient multi-GPU setups. This is crucial for training large AI models that require multiple graphics cards working together.
NVIDIA’s AI software stack, including CUDA, cuDNN, and TensorRT, provides optimized tools for every stage of AI development. This comprehensive approach makes development faster and more efficient.
Has AMD announced any upcoming AI-focused chips or technologies that could impact its market position?
AMD recently unveiled its MI300 series accelerators with promising performance metrics. These chips combine CPU and GPU elements specifically designed for AI workloads.
The company has announced plans to enhance its ROCm software platform with better support for popular frameworks like PyTorch and TensorFlow. This could address a major adoption barrier.
AMD is working on next-generation architecture improvements focused on AI-specific instructions and memory bandwidth. These developments aim to close the performance gap with NVIDIA in future products.